Science begins with the observation of an interesting pattern (e.g., “birds can fly”) or unexplained variation (e.g., “birds can fly but mammals can’t, except for bats”) and proceeds by testing different possible explanations, or hypotheses, for those patterns or variations. In the process of designing clear and convincing experiments that help to discriminate among many possible explanations, it is important to first identify exactly what aspect of the pattern/variation does each hypothesis hope to explain. In other words, it is important to identify which causal question is being asked and then identify any other possible answers (alternative hypotheses) that might also answer that question. A good experimental design will simultaneously test for all hypotheses so that the outcome of the experiment makes it clear that certain hypotheses are more likely than others.
This guide is meant to give you templates to help develop your own questions, hypotheses, and study designs (experiments and predictions) that will be well equipped to provide clear and compelling evidence for or against different explanations for patterns observed in nature.
Steps and Suggested Templates for Developing Questions, Hypotheses, Studies, and Predictions
- ✅ Start by identifying the causal question type (see Table 1 below).
- 🔍 Use the question template to frame your research question.
- 📊 Then write:
- a hypothesis (that explains what you expect and why): a general causal claim you will test.
- a prediction (based on your study design): a specific, measurable outcome expected in your proposed study.
Table 1: Types of Causal Questions and Their Components (Generalized for Biology, Medicine, and Applied Sciences) | ||||
Causal Type | Question Template | Typical Study Design | Hypothesis Format | Prediction Format |
Mechanistic (Proximate) | “How do/does [observed repeatable pattern] occur?” OR, if a factor has already been identified as being casually related to an outcome but the extent to which it does is unknown: “How does [identified factor] affect [outcome]?” OR, similarly: “How does [intervention, condition, or process] influence [biological or health outcome]?” | Controlled experiment or systems-based study | “Factor A causes outcome B.” | “Group exposed to A will show higher/lower B than control group.” |
Developmental (Ontogenetic) | “How does [trait, response, or condition] change over time or across life stages?” | Longitudinal or cross-sectional observational study | “Variable X affects how feature Y develops over time.” | “Y will increase/decrease or change pattern between early and late stages under X.” |
Functional (Adaptive) | “What is the benefit or purpose of [trait, behavior, treatment, or structure] in [context]?” | Applied or field-based outcome studies | “Feature or intervention F improves performance/survival in C.” | “Individuals with F will show better outcomes (e.g., lower risk, better recovery).” |
Evolutionary (Phylogenetic) | “How did [trait, feature, or condition] originate or diversify across lineages or populations?” | Comparative across populations or species | “T arose in response to evolutionary pressure P.” | “Lineages in environment E will more often exhibit trait T.” |
- ✏️ Use Table 2 (below) to help scaffold your thinking if you’re considering a comparative design.
Table 2: Comparative Designs in Causal Research | |||
Causal Type | Comparative Research Question | Template Hypothesis | Template Prediction |
Mechanistic (Proximate) | How does [treatment or exposure] affect [biological outcome] compared to [alternative condition]? | Individuals exposed to A will show different levels of outcome B than those exposed to C. | If the hypothesis is correct, Group A will have significantly higher B than Group C after 4 weeks. |
Developmental (Ontogenetic) | How does [trait or response] differ across [developmental stages or age groups]? | Trait B will increase (or change pattern) between early and late stages due to factor D. | Measurements of B will show a consistent upward trend across age groups from juvenile to adult. |
Functional (Adaptive) | How does [trait or behavior] contribute to [fitness, performance, or recovery] in different contexts? | Individuals with trait T will have higher fitness/success in context X than those without it. | In field trials, individuals with T will have 20% higher survival or task success over 30 days. |
Evolutionary (Phylogenetic) | How do patterns of [trait] differ across lineages or environments? | Species in environment E will more often possess trait T than those in environment F. | In a sample of 20 species from each environment, the presence of T will be twice as common in E. |
- If you’re having trouble identifying your question, hypotheses, and the best designs for testing those hypotheses, ask yourself:
- What kind of question am I trying to answer?
- What are plausible explanations (hypotheses)?
- What kind of data would support or refute them?
- What kind of experiment would generate those data?
- What specific outcome (prediction) would I expect in that design if the hypothesis is true?
Bonus Diagnostic Prompts (to check for common mistakes)
- ❌ If your “hypothesis” includes a specific number or outcome, it might actually be a prediction.
- ❌ If your “question” includes the word “will,” it may be phrased as a hypothesis or prediction instead.
- ❌ If your “prediction” simply rewords the hypothesis vaguely, try specifying what will be measured and when.
- ❓ If your hypothesis doesn’t claim a relationship or cause, it may be descriptive or incomplete.
- 🔎 If you’re asking “why” or “how,” you’re probably aiming for a causal question.
Additional Tips
- Hypotheses are general explanations; predictions are testable outcomes based on those explanations.
- Use comparative language when designing experiments (e.g., “compared to,” “relative to”) to anchor predictions.
- When in doubt, write out:
- Question → Hypothesis → Experimental Design → Variables (Independent Variables, Dependent Variables) → Prediction (about Dependent Variables)
- If you’re having trouble identifying your question, hypotheses, and the best designs for testing those hypotheses, ask yourself:
- What kind of question am I trying to answer?
- What are plausible explanations (hypotheses)?
- What kind of data would support or refute them?
- What kind of experiment would generate those data?
- What specific outcome (prediction) would I expect in that design if the hypothesis is true?
- Consider how the design of your study (cross-sectional, longitudinal, experimental) constrains the kinds of questions and predictions you can ask.
- Use these tables as a checklist before submission of research proposals (or even results write-ups) to ensure logical alignment across all elements.
- If you’re having trouble identifying your question, hypotheses, and the best designs for testing those hypotheses, ask yourself:
- Question → Hypothesis → Experimental Design → Variables (Independent Variables, Dependent Variables) → Prediction (about Dependent Variables)
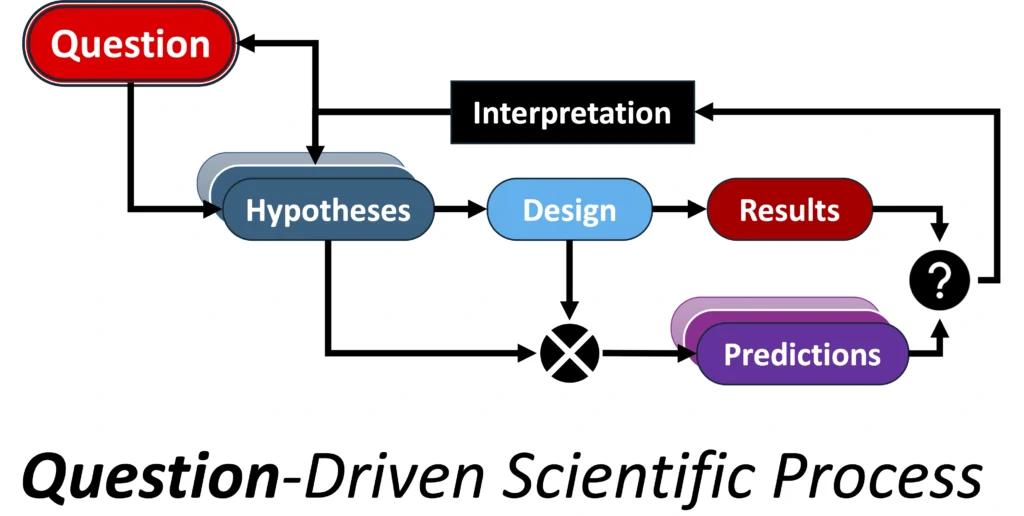